Abstract
With the rapid evolution in Large Language Models, multimodal large language models have recently achieved impressive vision-language understanding, reasoning, and interaction capabilities through visual instruction tuning. In this talk, we firstly introduce a mask-text instruction tuning approach Osprey, to extend MLLMs by incorporating fine-grained mask regions into language instruction, aiming at achieving pixel-wise visual understanding. Secondly, visual projector plays a crucial role to bridge the vision and language model while a simple MLP may not effectively preserve all visual contexts via one-to-one transformation. To generate the condensed visual tokens, we present a visual projector with a coarse-to-fine scheme to inject the enriched characteristics. It compresses the visual tokens by 75%~89% while achieving comparable or even better performance across diverse benchmarks with significantly higher efficiency.
Speaker Bio
Jianke Zhu received the master degree from University of Macau in Electrical and Electronics Engineering, and the PhD degree in computer science and engineering from The Chinese University of Hong Kong, Hong Kong in 2008. He held a post-doctoral position at the BIWI Computer Vision Laboratory, ETH Zurich, Switzerland. He is currently a Professor with the College of Computer Science, Zhejiang University, Hangzhou, China. His research interests include computer vision and robotics. He is a senior member of IEEE.
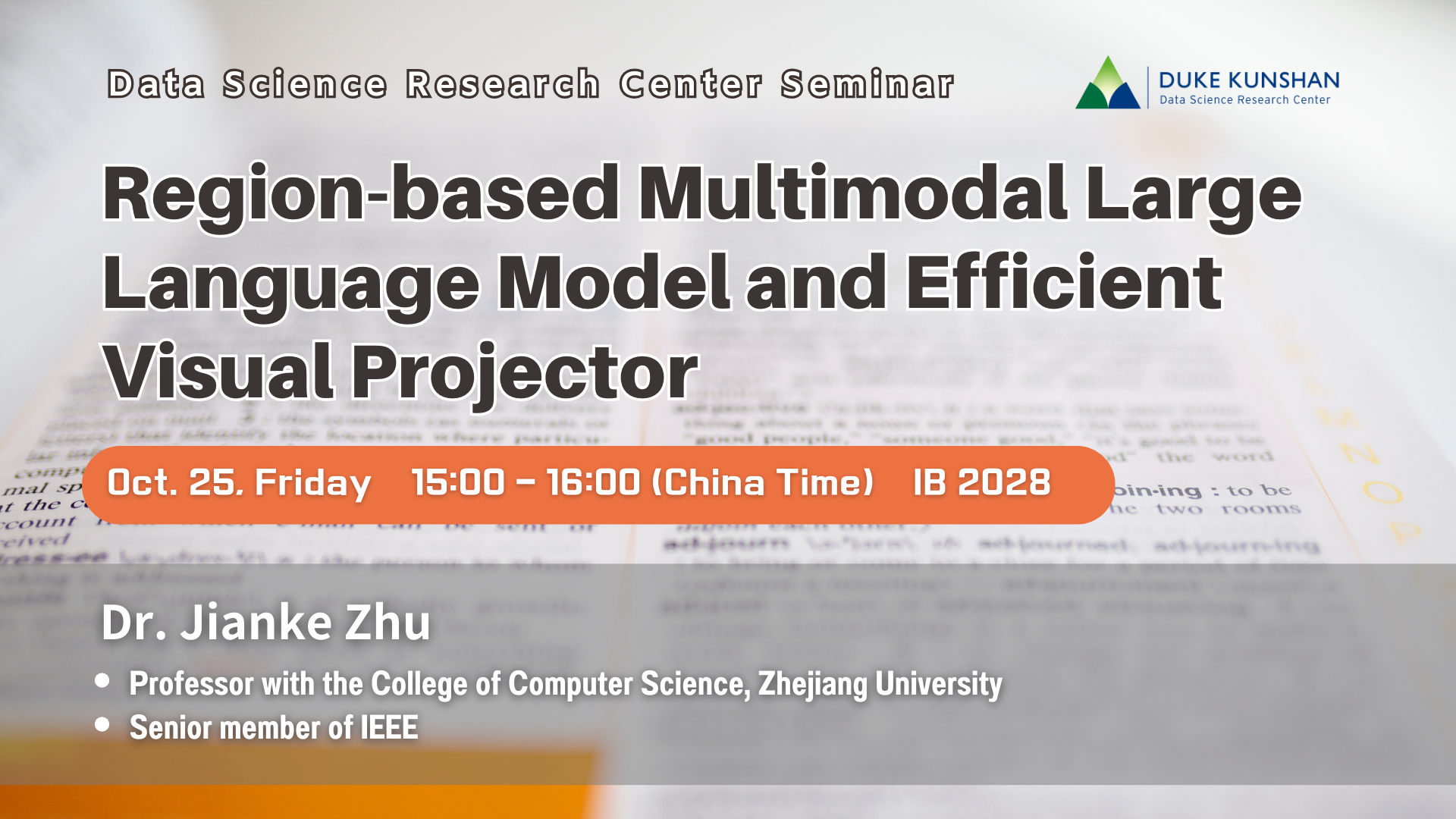