Abstract:
Few-shot image classification aims to quickly adapt neural networks to new classification tasks with a few shots, where a distribution shift in categories, domains or class granularities usually appears. This task distribution shift brings a research problem of how to deal with knowledge transfer between the support classes and the query class. In this talk, I will introduce several recent researches for few-shot learning. Firstly, we observe that knowledge learned from image background at training may be harmful for few-shot evaluation at test time. This finding identifies an important characteristic of few-shot classification that distinguishes itself from normal classification tasks. To alleviate the bias introduced by the backgrounds, we design a framework called COSOC. Secondly, we discuss the channel bias problem in image representations that exists in task distribution shift at test-time. We present algorithms to solve these feature bias problems, which correct the biased representations of a given test image by exploiting useful information of the class in the training set that is most relevant to the test image.
Bio:
Dr. Zenglin Xu is a professor at Harbin Institute of Technology Shenzhen and he is also affiliated with Peng Cheng Lab.
He received the Ph.D. degree in computer science and engineering from the Chinese University of Hong Kong. He has been working at Michigan State University, Cluster of Excellence at Saarland University and Max Planck Institute for Informatics, Purdue University, and University of Electronic Science and Technology of China.
Dr. Xu’s research interests include machine learning and its applications in computer vision, natural language processing, and health informatics. He is a board of governor of INNS. He currently serves as an associate editor to Neural Networks and Neurocomputing. He is the recipient of the outstanding student paper honorable mention of AAAI 2015, the best student paper runner up of ACML 2016, and the 2016 young researcher award from APNNS.
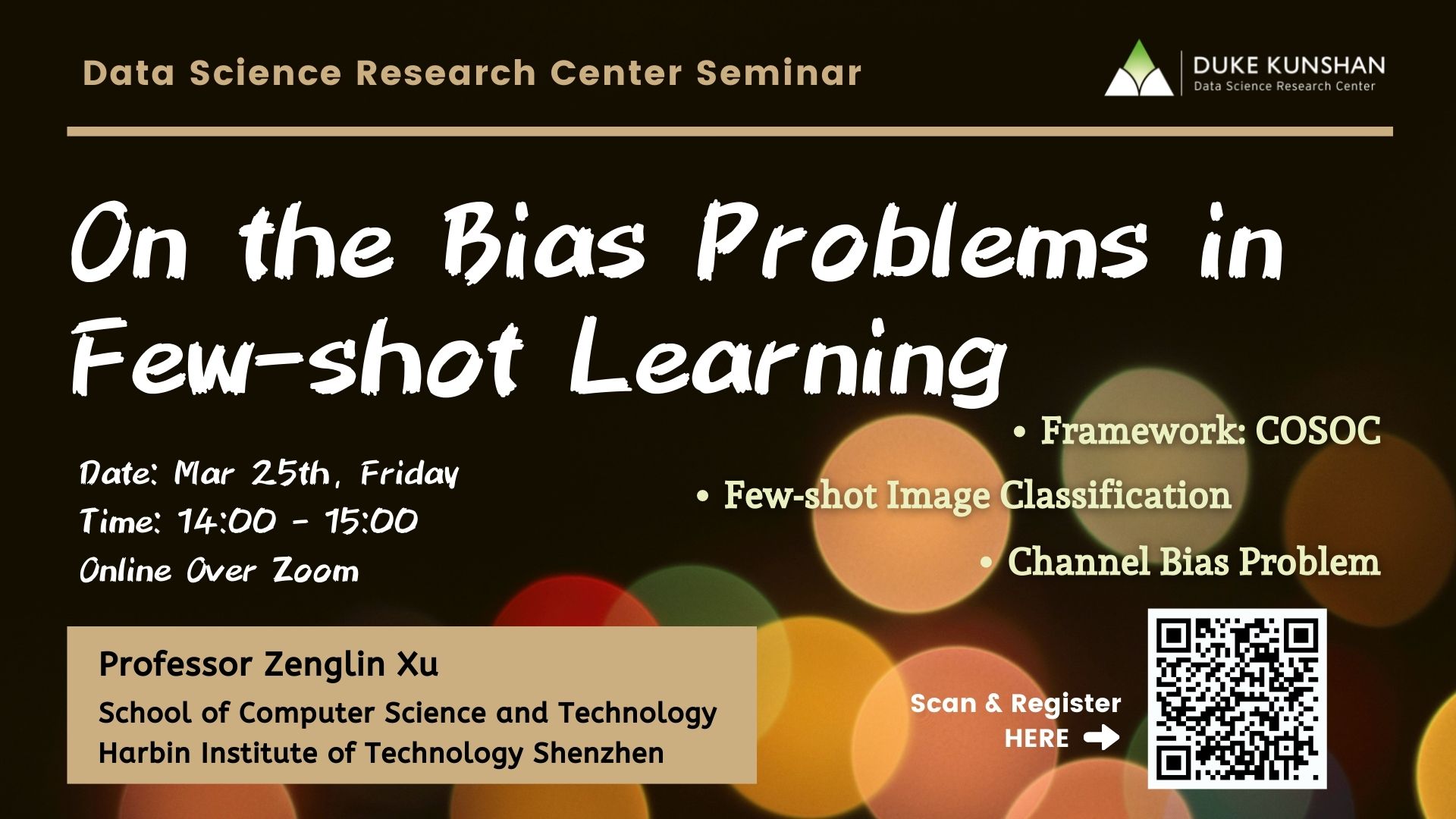