Abstract:
The Segment Anything Model (SAM) has demonstrated outstanding adaptation to medical image segmentation but still faces three major challenges. Firstly, the huge computational costs of SAM limit its real-world applicability. Secondly, SAM depends on manual annotations (e.g., points, boxes) as prompts, which are laborious and impractical in clinical scenarios. Thirdly, SAM handles all segmentation targets equally, which is suboptimal for diverse medical modalities with inherent heterogeneity. To address these issues, we propose a universal medical image segmentation. Extensive experiments indicate that our approach outperforms state-of-the-arts in diverse medical imaging segmentation takes, displaying superior zero-shot learning and modality transfer ability. Especially, our framework uses only 31.4% parameters compared to SAM-Base.
Biography:
Professor Xiangjian (Sean) He is a National Talent, and in list of the ‘World Top 2% Scientists’ reported by Stanford Universiy and Elsevier in 2022, 2023, 2024etc. He is currently the Deputy Head of Computer Science School, the Lead of Research Groups of the Faculty of Science and Engineering and the Director of Computer Vision and Intelligent Perception Laboratory at the University of Nottingham, Ningbo, China (UNNC). He has been responsible for and hosted a number of Australian national ARC projects, including a major national youth science and technology project (ARC-Discovery for early career researchers), a major national science and technology project (ARC-Discovery), and two major national joint innovation science and technology projects (ARC-Linkage). He also participated in five China Natural Science Foundation projects and two Hong Kong Research Foundation projects as a core member.
He was an International Advisor for the Journal of the Hong Kong Institution of Engineers, an associate editor of journals such as HCCIS and SNCS, an editorial board member of several SCI journals, and a guest editor of journals such as IEEE Transactions and Elsevier journals. He is an International Expert of the Australian Research Council.
He was the Professor of Computer Science and the Leader of Computer Vision and Pattern Recognition Laboratory at the Global Big Data Technologies Centre (GBDTC) at the University of Technology Sydney (UTS) from 2011-2022. He led the UTS and Hong Kong Polytechnic University (PolyU) joint research project teams winning the 1st Runner-Up prize for the 2017 VIP Cup, and the champion for the 2019 VIP Cup, awarded by IEEE Signal Processing Society.
He has been carrying out research mainly in the areas of computer vision, data analytics and machine learning in the previous years. He has recently been leading his research teams for deep-learning-based research for various applications.
He has published many papers including ESI highly cited papers in high-end journals of Journal of the Association for Information Science and Technology, ACM Computing Surveys, IEEE Transactions (such as TPAMI, TII, TDSC, TNSE, TMC, TPDS, TMM, TC, TCSVT, TITS, TCC, TR, TCE, etc.) and Elsevier (such as PR, SP, AC, IS, FGCS, Neurocomputing, CN, CSS, NCP, etc.), as well as in top conferences such as AAAI, ACL, IJCAI, CVPR, ECCV, ACM MM, WACV, etc.
He has played various chair roles in many international conferences such as ACM MM, MMM, ICDAR, IEEE BigDataSE, IEEE BigDataService, IEEE TrustCom, IEEE CIT, IEEE AVSS, IEEE ICPR and IEEE ICARCV.
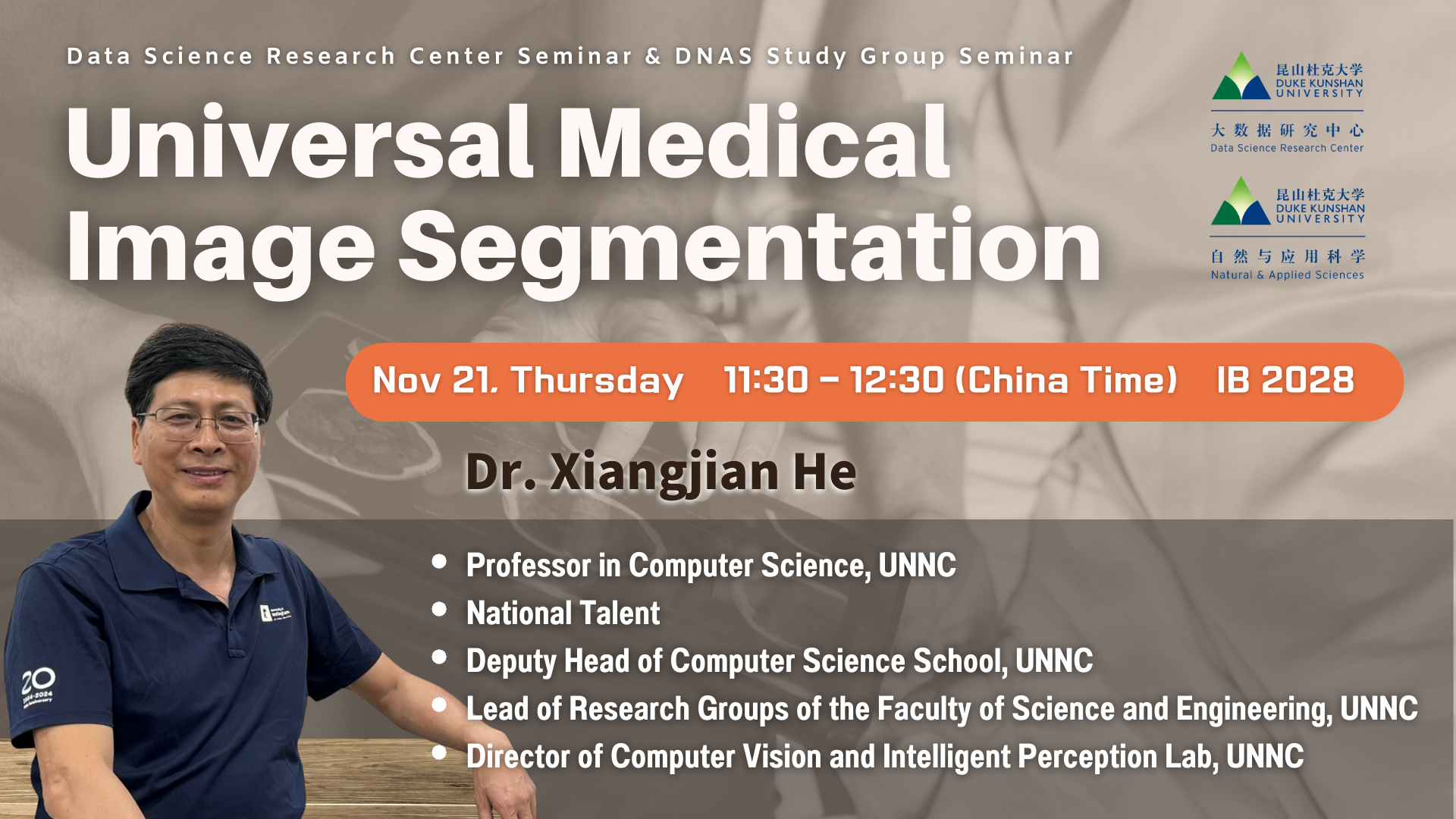